Learn2Reg 2020 Archive
On this site, you find all the important information about last years Learn2Reg challenge:
- Results
- Materials and Presentations
- Sponsors and Prizes
- Datasets
Results
The numerical results and ranks for all four tasks.
Task 1:
Task 2:
Task 3:
Task 4:
Overview:
Materials
MICCAI Pathable Link: https://miccai2020.pathable.co/meetings/virtual/4d3PHdZEujMPfPhoy
Direct link to workshop slides: https://cloud.imi.uni-luebeck.de/s/nAgNDSj9J2GEX6d
Presentations of challenge participants
Tony Mok: https://cloud.imi.uni-luebeck.de/s/C9epKzJsCXR47q3
Niklas Gunnarsson: -
Marek Wodzinski: https://cloud.imi.uni-luebeck.de/s/xR7wREKTWwQCapn
Théo Estienne: https://cloud.imi.uni-luebeck.de/s/FJ3szqokbZRfjzj
Samuel Joutard: -
Fraunhofer MEVIS: https://cloud.imi.uni-luebeck.de/s/CkdBRqoFBx3mCjY
Constance Fourcade: -
Lasse Hansen: https://cloud.imi.uni-luebeck.de/s/J4f9pYcAYs4yfHE
Prizes
NVIDIA is sponsoring a brand new TITAN RTX (retail price: 2500 $) for the overall challenge winner (all 4 tasks). For more details on the GPU, please see here: https://www.nvidia.com/en-us/deep-learning-ai/products/titan-rtx/
We are very happy to announce that Scaleway is sponsoring a 500 € prize (in GPU computing) for the best GPU-based submission (inclusive of time bonus). That's 500 hours or a full month of 16GB GPU cloud computing at https://www.scaleway.com/en/gpu-instances/
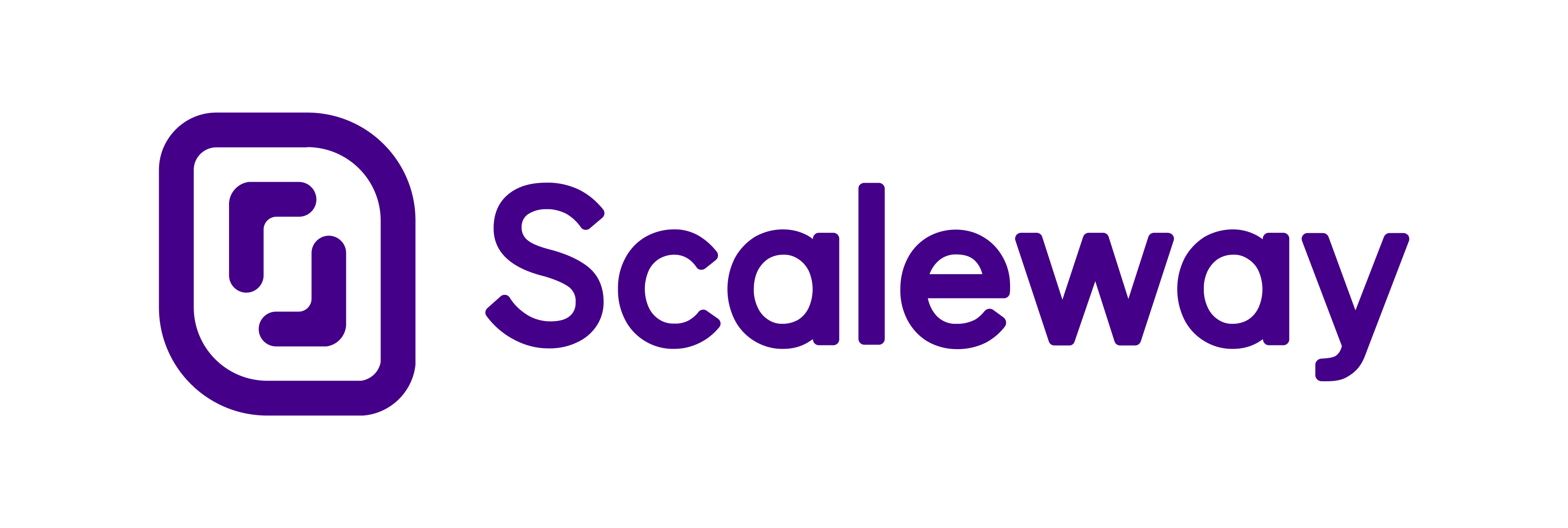
Datasets:
Task1:
Training/Validation: Download | pairs_val.csv
Test: Download | pairs_val.csv
Description: The database contains 22 subjects with low-grade brain gliomas and is intended to help develop MRI vs. US registration algorithms to correct tissue shift in brain tumor resection. For the task, we included the T1w and T2-FLAIR MRIs, and spatially tracked intra-operative ultrasound volumes taken after craniotomy and before resection started. Matching anatomical landmarks were annotated between T2FLAIR MRI and 3D ultrasound volumes to help validate registration algorithm accuracy. All scans were acquired for routine clinical care of brain tumor resection procedures at St Olavs University Hospital (Trondheim, Norway). For each clinical case, the pre-operative 3T MRI includes Gadolinium-enhanced T1w and T2 FLAIR scans, and the intra-operative US volume was obtained to cover the entire tumor region after craniotomy but before dura opening, as well as after resection was completed. A detailed user manual is included in the data package download.
Number of cases: Training: 22, Test: 10
Annotations: Matching anatomical landmarks were annotated between T2FLAIR MRI and 3D ultrasound volumes. The reference anatomical landmarks were selected by Rater 1 in the ultrasound volume before dura is open after craniotomy. Then two raters selected matching anatomical landmarks in the ultrasound volumes obtained after tumor resection using the software 'register' from MINC Toolkit. The ultrasound landmark selection was repeated twice for each rater with a time interval of at least one week. Finally, the results (4 points for each landmark location) were averaged. Eligible anatomical landmarks include deep grooves and corners of sulci, convex points of gyri, and vanishing points of sulci. Same raters produced the anatomical landmarks for both the training and testing data. To help with deep learning purposes, the landmarks have also been voxelized as spheres in the same 3D space as the MRI/US scans. In addition, the image header transforms are also provided separately for the users.
Pre-Processing: All MRI scans were corrected for field inhomogeneity, and T1w MRI is rigidly registered to T2FLAIR MRI. For each subject, the MRI and 3D ultrasound volumes were resampled to the same space and dimension (256x256x288) at an isotropic ~0.5mm resolution.
Non-disclosure Agreement: Unlike the training data, which were publicly released as the EASY-RESECT database, the CuRIOUS 2020 for Learn2Reg Challenge test data are not public. They cannot be shared and used for any purpose but the challenge evaluation.
Citation: Y. Xiao, M. Fortin, G. Unsgård , H. Rivaz, and I. Reinertsen, “REtroSpective Evaluation of Cerebral Tumors (RESECT): a clinical database of pre-operative MRI and intra-operative ultrasound in low-grade glioma surgeries”. Medical Physics, Vol. 44(7), pp. 3875-3882, 2017.
Task2:
Training/Validation: Download | pairs_val.csv
Test: Download | pairs_val.csv
Description: The database consists of 60 3D HRCT thorax images taken from 30 subjects. For each subject, an inspiration and expiration scan is taken. The data was gathered from the Department of Radiology at the Radboud University Medical Center, Nijmegen, The Netherlands. One additional challenge with this data is that the lungs are not fully visible in the expiration scans.
Number of cases: Training: 20, Test: 10
Annotation: For all scans, an automatic lung segmentation is provided. For evaluating the registration methods, we use manually annotated landmarks. We also plan to provide automatically computed keypoint correspondences for all training pairs (these are considered noisy labels with residual errors of 1-2mm).
Pre-processing: Common pre-processing to same voxel resolutions and spatial dimensions as well as affine pre-registration will be provided to ease the use of learning-based algorithms for participants with little prior experience in image registration.
Citation: Hering, Alessa, Murphy, Keelin, & van Ginneken, Bram. (2020). Learn2Reg Challenge: CT Lung Registration - Training Data [Data set]. Zenodo. http://doi.org/10.5281/zenodo.3835682; Hering, Alessa, Murphy, Keelin, & van Ginneken, Bram. (2020). Learn2Reg Challenge: CT Lung Registration - Test Data [Data set]. Zenodo. http://doi.org/10.5281/zenodo.4048761
Task3:
Training/Validation: Download | pairs_val.csv
Test: Download | pairs_val.csv
Description: All scans were captured during portal venous contrast phase with variable volume sizes (512 × 512 × 53 ~ 512 × 512 × 368) and field of views (approx. 280 × 280 × 225 mm3 ~ 500 × 500 × 760 mm3). The in-plane resolution varies from 0.54 × 0.54 mm2 to 0.98 × 0.98 mm2, while the slice thickness ranged from 1.5 mm to 7.0 mm.
Number of cases: Training: 30, Test: 20
Annotation: Thirteen abdominal organs were considered regions of interest (ROI), including spleen, right kidney, left kidney, gall bladder, esophagus, liver, stomach, aorta, inferior vena cava, portal and splenic vein, pancreas, left adrenal gland, and right adrenal gland. The organ selection was essentially based on [Shimizu A, Ohno R, Ikegami T, Kobatake H, Nawano S, Smutek D. Segmentation of multiple organs in non-contrast 3D abdominal CT images. International Journal of Computer Assisted Radiology and Surgery. 2007;2:135–142.]. As suggested by a radiologist, the heart was excluded for lack of full appearance in the datasets, and instead the adrenal glands were included for clinical interest. These ROIs were manually labeled by two experienced undergraduate students with 6 months of training on anatomy identification and labeling, and then verified by a radiologist on a volumetric basis using the MIPAV software.
Pre-processing: Common pre-processing to same voxel resolutions and spatial dimensions as well as affine pre-registration will be provided to ease the use of learning-based algorithms for participants with little prior experience in image registration.
Citation: Xu, Zhoubing et al. Evaluation of six registration methods for the human abdomen on clinically acquired CT, IEEE Transactions on Biomedical Engineering, 63 (8), pages=1563-1572, 2016
Task4:
Training/Validation: Download | pairs_val.csv
Test: Download | pairs_val.csv
Description: The dataset consisted of MRI acquired in 90 healthy adults and 105 adults with a non-affective psychotic disorder (56 schizophrenia, 32 schizoaffective disorder, and 17 schizophreniform disorder) taken from the Psychiatric Genotype/Phenotype Project data repository at Vanderbilt University Medical Center (Nashville, TN, USA). Patients were recruited from the Vanderbilt Psychotic Disorders Program and controls were recruited from the surrounding community. The MRI data will show parts of the brain covering the hippocampus formation. The algorithm targets the alignment of two neighboring small structures (hippocampus head and body) with high precision on mono-modal MRI images between different patients (new insights into learning based registration due to a large-scale dataset). All images were collected on a Philips Achieva scanner (Philips Healthcare, Inc., Best, The Netherlands). Structural images were acquired with a 3D T1-weighted MPRAGE sequence (TI/TR/TE, 860/8.0/3.7 ms; 170 sagittal slices; voxel size, 1.0 mm3). The data is provided by the Vanderbilt University Medical Center (VUMC) and part of the medical segmentation decathlon.
Number of cases: Training: 263, Test: 131
Annotation: We use anatomical segmentations (and deformation field statistics) to evaluate the registration. Manual tracing of the head, body, and tail of the hippocampus on images was completed following a previously published protocol [Pruessner, J. et al. Volumetry of hippocampus and amygdala with high- resolution MRI and three-dimensional analysis software: minimizing the discrepancies between laboratories. Cerebral cortex 10, 433–442 (2000).; Woolard, A. & Heckers, S. Anatomical and functional correlates of human hippocampal volume asymmetry. Psychiatry Research: Neuroimaging 201, 48–53 (2012).]. For the purposes of this dataset, the term hippocampus includes the hippocampus proper (CA1-4 and dentate gyrus) and parts of the subiculum, which together are more often termed the hippocampal formation [Amaral, D. & Witter, M. The three-dimensional organization of the hip- pocampal formation: a review of anatomical data. Neuroscience 31, 571– 591 (1989)]. The last slice of the head of the hippocampus was defined as the coronal slice containing the uncal apex.
Pre-Processing: Common pre-processing to same voxel resolutions and spatial dimensions will be provided to ease the use of learning-based algorithms for participants with little prior experience in image registration.
Citation: A Simpson et al.: "A large annotated medical image dataset for the development and evaluation of segmentation algorithms" arXiv 2019
Submission
The Learn2Reg challenge has an automatic evaluation system for validation scans running on grand-challenge.org. You can submit your deformation fields as zip file at the Create Challenge Submission page and results for each task will be published on the validation leaderboard (note that this does not reflect the final ranking as test scans are different and ranks will be computed based on significance, weighted scores, etc.). Docker submissions have to be sent as download links to learn2reg@gmail.com. Test set deformation fields can also be sent as download links via mail or as submission to the test leaderboard (note that no results will be published before the challenge deadlines).
Submission Format
Submissions must be uploaded as zip file containing displacement fields (displacements only, identity grid is added) for all validation pairs for all tasks (even when only participating in a subset of the tasks, in that case submit deformation fields of zeroes for all remaining tasks). You can find the validation pairs for each task as CSV files at the Datasets page. The convention used for displacement fields depends on scipy's map_coordinates() function, thus expecting displacement fields in the format [[x, y, z], X, Y, Z], where x, y, z and X, Y, Z represent voxel displacements and image dimensions, respectively. The evaluation script expects .npz files using half-precision format ('float16') and having shapes 3x128x128x144 for task 1 (half resolution), 3x96x96x104 for task 2 (half resolution), 3x96x80x128 for task 3 (half resolution) and 3x64x64x64 (full resolution) respectively. The file structure of your submission should look as follows:
The first four digits represent the case id of the fixed image (as specified in the corresponding pairs_val.csv) with leading zeros, the second four digits represent the case id of the moving image. For the paired registration tasks the fixed and moving image are defined as MR and US (task 1) and exhale and inhale scan (task 2) respectively. Note that in conventional lung registration tasks the exhale scan is registered to the inhale scan. However, in this dataset the field-of-view for the exhale scan is partially cropped which leads to missing correspondences in the inhale scan. We further provide a python script to create a submission zip file from a folder of uncompressed, full precision (float32) and full resolution (image resolution) deformation fields (same file structure as above): create_submission.py (zero deformation fields output: submission.zip). If you have any problems with your submissions or find errors in the evaluation code (see below), please contact Adrian Dalca, Alessa Hering, Lasse Hansen and Mattias Heinrich at learn2reg@gmail.com.
Note for PyTorch users: When using PyTorch as deep learning framework you are most likely to transform your images with the grid_sample() routine. Please be aware that this function uses a different convention than ours, expecting displacement fields in the format [X, Y, Z, [z, y, x]] and normalized coordinates between -1 and 1. Prior to your submission you should therefore convert your displacement fields to match our convention (see above).
Metrics and Evaluation
Since registration is an ill-posed problem, the following metrics will be used to determine per case ranks between all participants
- TRE: target registration error of landmarks (Tasks 1, 2)
- DSC: dice similarity coefficient of segmentations (Tasks 3, 4)
- DSC30: robustness score (30% lowest DSC of all cases) (Tasks 3, 4)
- HD95: 95% percentile of Hausdorff distance of segmentations (Tasks 3, 4)
- SDlogJ: standard deviation of log Jacobian determinant of the deformation field (Tasks 1, 2, 3, 4)
DSC measures accuracy; HD95 measures reliability; Outliers are penalised with the robustness score (DSC30: 30% of lowest mean DSC); The smoothness of transformations (SD of log Jacobian determinant) are important in registration, see references of Kabus and Low. For final evaluation on test sets all metrics but robustness (DSC30) use mean rank per case (ranks are normalised to between 0.1 and 1, higher being better). For multi-label tasks the ranks are computed per structure and later averaged. As done in the Medical Segmentation Decathlon we will employ "significant ranks" http://medicaldecathlon.com/files/MSD-Ranking- scheme.pdf. Across all metrics an overall score is aggregated using the geometric mean. This encourages consistency across criteria. Missing results will be awarded the lowest rank (potentially shared and averaged across teams). For further insights into the used metrics and evaluation routines we provide the evaluation script that is running on the automatic evaluation system: evaluation.py
References:
- AD Leow, et al.: "Statistical properties of Jacobian maps and the realization of unbiased large-deformation nonlinear image registration" TMI 2007
- S Kabus, et al.: "Evaluation of 4D-CT Lung Registration" MICCAI 2009